How to implement Digital twin technology in your factory
The recent advent of IOT and Industry 4.0 has introduced new terminology in the field of manufacturing and mechanical engineering. One such term is Digital twin.
After reading this article you will be in a position to answer the following questions
- What is Digital Twin?
- Is Digital Twin and Digital Thread the same?
- What is the history of Digital Twin?
- How digital twin can be implemented in my factory?
The Digital Twin Concept
While the terminology has changed over time, the basic concept of the Digital Twin model has remained fairly stable from its inception in 2002. It is based on the idea that a digital informational construct about a physical system could be created as an entity on its own. This digital information would be a “twin” of the information that was embedded within the physical system itself and be linked with that physical system through the entire lifecycle of the system.
Origins of “the Digital Twin Concept”
The concept of the Digital Twin dates back to a University of Michigan presentation to industry in 2002 for the formation of a Product Lifecycle Management (PLM) center. The figure was simply called “Conceptual Ideal for PLM and was originated by Dr Grieves”. However, it did have all the elements of the Digital Twin: real space, virtual space, the link for data flow from real space to virtual space, the link for information flow from virtual space to real space, and virtual sub-spaces.
The premise driving the model was that each system consisted of two systems, the physical system that has always existed and a new virtual system that contained all of the information about the physical system. This meant that there was a mirroring or twinning of systems between what existed in real space to what existed in virtual space and vice versa
Digital twin and digital thread
The digital twin and digital thread are not similar concepts. Both these concepts help in understanding the actual working of the physics-based models by reflecting the exact operating conditions like performance and failure modes, but their application and functioning is different from one another. Let’s take a detailed look at both of these concepts.
The main goal of a digital twin is to create, build, and test your equipment in a virtual environment. Only when you get to understand your product’s functionality, and how it performs to your requirements, would you physically manufacture it. Digital twin comprises of several elements like manufacturing simulations, 3D CAD models, and real-time data feeds from sensors that are incorporated into the physical operating environment. Digital twin can benefit all aspects of manufacturing, right from design to real-time data feed. The aim of creating a digital twin is not just to cut prototyping or construction costs, but also to predict failures more easily and accurately, and effectively, thereby reducing both maintenance costs and downtime. For example, the digital twin created for a wind farm helps in informing the manufacturer about the configuration of each wind turbine prior to procurement and construction.
The digital thread consists of a communication framework that helps in facilitating an integrated view and connected data flow of the product’s data throughout its lifecycle. The digital thread concept helps in delivering the right information at the right time and at the right place. Digital thread provides the ability of accessing, integrating, analysing, and transforming data from disparate systems into actionable information throughout the product lifecycle. By developing digital thread, product engineers can collaborate with manufacturing engineers for creating a 3D model linked to visuals for production process instructions.
Digital twin implementation
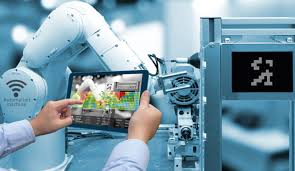
The actual implementation of a digital twin depends on the intended business outcome and sophistication of business logic. Different integration scenarios for Digital Twin Implementation include.
Twin-to-device integration: The physical object needs to be securely connected and managed. Onboarding established a relationship to an instance. This may happen before installation (e.g. during configuration or production) or after installation (in a two-phased approach with certificates being pre-installed earlier). Streams or batches of live data often require protocol conversion, semantic mapping, and transformation before being ingested into a big data store infrastructure. This allows querying object state and historic information captured as time series.
Twin-to-twin integration: As an optional component, an integration to a digital twin managed by a service provider (e.g. a telematics vendor) or by a supplier (e.g. by an automation equipment vendor) may be needed if the physical object is not managed by the provider of the digital twin.
Twin-to-system-of-record integration: Integration with business information and engineering systems provides essential context along the lifecycle of the physical object
- PLM for engineering bill of material, components and spare parts, software versioning (for embedded systems)
- CAD/CAM/CAE for 2D and 3D models, layouts, assembly information
- Manufacturing systems for product traceability, serialization, manufacturing bill of material
- ERP for product variants, financial information (e.g. depreciation), equipment and spare parts inventory
- ERP/CRM and supplier networks for service contracts, business partners and roles, SLAs
Twin-to-system-of-intelligence integration: Most digital twins are not consumed directly by end-users, but interact with systems of intelligence through events and notifications while exposing condition monitoring and historic information; rule handling, data science algorithms, and machine learning create insight from streams of live data (e.g. anomaly detection, issue segmentation, health scores) and provide predictions on the future state (e.g. remaining lifetime, time of arrival forecasts)
The digital twin implementation will largely be managed from the cloud to facilitate the network-centric engagement models described above. However, most scenarios will distribute actual data and algorithms between an edge or gateway implementation (located on or near the physical object) and the cloud in a distributed architecture. Learning and model development are primary functions in the cloud, however, not all data is relevant to be transmitted. In many cases, only change information and events will be sent into the cloud as a stream while data locally and temporally persisted can be replicated to resolve underlying issues and to evolve algorithms.